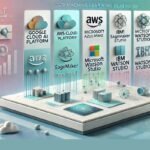
Top ML Platforms for Seamless Integrations
November 14, 2024
Top 5 Machine Learning Platforms Compared: Machine learning platforms are essential tools for digital marketing professionals, developers, and content creators. As machine learning plays a growing role in predictive analytics, customer behavior analysis, and content personalization, choosing the right platform is critical. This article compares the top five options, providing insights to help you make an informed decision.
Table of Contents
1. Google Cloud AI Platform
Google Cloud AI Platform stands out with its vast ecosystem, high-performance infrastructure, and scalability. Ideal for enterprises and businesses looking for a comprehensive machine learning solution, this platform offers extensive tools and integrations with Google’s cloud services.
Features and Benefits of Google Cloud AI Platform
- Comprehensive ML Toolkit: Includes tools for data preprocessing, model training, and deployment.
- AutoML Capabilities: Supports automated machine learning for faster model creation.
- Integration with BigQuery: For advanced data analytics, Google’s BigQuery is seamlessly integrated.
Pros and Cons of Google Cloud AI Platform
Pros
- Scalable solutions suitable for large data sets.
- AutoML tools for faster time-to-market.
- Strong security and compliance features.
Cons
- Costs can scale with large datasets and computational power.
- Advanced features may require technical expertise.
2. Microsoft Azure Machine Learning
Microsoft Azure Machine Learning is a versatile platform offering advanced ML and AI tools. It’s designed for developers, data scientists, and IT professionals, making it a robust option for end-to-end machine learning workflows.
Key Features of Microsoft Azure Machine Learning
- Drag-and-Drop Interface: Simplifies model building with minimal code.
- Integration with Azure Services: Connects with Microsoft services, including Power BI for analytics and visualization.
- Support for Multiple Languages: Azure supports Python, R, and other programming languages.
Advantages and Disadvantages of Microsoft Azure Machine Learning
Advantages
- Intuitive interface suitable for beginners and advanced users.
- Strong support for collaborative projects.
- Provides flexibility with customizable resources.
Disadvantages
- High costs for extensive usage and storage.
- Some features are locked behind enterprise-level pricing.
3. Amazon SageMaker
Amazon SageMaker is one of the most popular machine learning platforms, providing a flexible environment for building, training, and deploying machine learning models. Known for its efficiency, SageMaker appeals to developers and businesses looking for cloud-based solutions.
Amazon SageMaker’s Unique Features
- Fully Managed ML Service: Allows users to focus on model development without handling infrastructure.
- Built-In Algorithms and Frameworks: Supports a range of pre-built algorithms and frameworks.
- Ground Truth Labeling: Assists with creating labeled datasets for supervised learning.
Pros and Cons of Amazon SageMaker
Pros
- Flexibility in model training with built-in or custom algorithms.
- Access to Amazon’s high-quality infrastructure and security.
- Ground Truth labeling feature saves time for large-scale labeling tasks.
Cons
- Pricing may vary significantly depending on model complexity.
- Steeper learning curve for beginners.
4. IBM Watson Studio
IBM Watson Studio combines AI and machine learning with data science tools, ideal for companies focused on advanced analytics and AI-driven projects. IBM Watson is known for its cognitive computing capabilities, making it a valuable asset for data-intensive projects.
Notable Features of IBM Watson Studio
- Comprehensive Data Science Toolkit: Includes SPSS, AutoAI, and Data Refinery.
- Collaborative Environment: Supports teamwork with tools for version control and resource sharing.
- Natural Language Processing (NLP): Advanced NLP capabilities for text and speech analysis.
Pros and Cons of IBM Watson Studio
Pros
- Powerful for businesses focused on AI research and innovation.
- High level of customization for advanced users.
- Strong security and compliance for regulated industries.
Cons
- Limited third-party integrations outside IBM’s ecosystem.
- Higher price point for enterprise features.
5. DataRobot
DataRobot is an end-to-end machine learning platform, specializing in automated machine learning (AutoML). Known for its ease of use and automated model selection, DataRobot caters to professionals who want to accelerate their ML projects.
Key Benefits of DataRobot
- Automated Model Building: Simplifies the ML process with AutoML for rapid deployment.
- Explainable AI: Offers model insights and interpretability, making it easy to understand predictions.
- Rapid Deployment: Streamlined process from data ingestion to model deployment.
Pros and Cons of DataRobot
Pros
- User-friendly with minimal coding needed.
- High-speed model training and deployment.
- Suitable for professionals without a strong ML background.
Cons
- Limited customization for advanced data scientists.
- May lack flexibility compared to traditional ML platforms.
Top 5 Machine Learning Platforms Compared: A Comprehensive Recap
Platform | Key Features | Pros | Cons |
---|---|---|---|
Google Cloud AI Platform |
– AutoML capabilities – Integration with BigQuery – Comprehensive ML toolkit |
– Scalable for large data sets – Strong security features – AutoML for faster model creation |
– Expensive for large datasets – Requires technical expertise |
Microsoft Azure Machine Learning |
– Drag-and-drop interface – Integration with Azure services – Multi-language support (Python, R, etc.) |
– User-friendly for beginners – Collaborative project support – Flexible resource customization |
– High costs for extensive use – Some features locked behind enterprise pricing |
Amazon SageMaker |
– Fully managed ML service – Built-in algorithms and frameworks – Ground Truth labeling |
– Flexible model training – High-quality infrastructure – Efficient for large-scale labeling |
– Variable pricing – Steep learning curve for beginners |
IBM Watson Studio |
– Advanced NLP capabilities – Comprehensive data science toolkit – Collaborative environment |
– Powerful for AI research – High customization options – Strong security for regulated industries |
– Limited third-party integrations – Expensive enterprise features |
DataRobot |
– AutoML for rapid deployment – Explainable AI – Streamlined data-to-deployment process |
– User-friendly with minimal coding – High-speed model training – Ideal for non-experts |
– Limited customization for experts – Less flexible than traditional platforms |
Frequently Asked Questions
What is the best machine learning platform for beginners?
For beginners, Microsoft Azure Machine Learning is often recommended due to its user-friendly drag-and-drop interface. Amazon SageMaker’s Ground Truth tool and DataRobot’s AutoML features are also beginner-friendly, offering a more streamlined experience.
Which machine learning platform offers the best scalability?
Google Cloud AI Platform and Amazon SageMaker are both highly scalable, making them ideal for large-scale projects. Their robust cloud infrastructures can handle extensive data processing and model training without compromising performance.
Is IBM Watson Studio worth the investment for small businesses?
IBM Watson Studio is powerful but may be better suited for larger organizations or those needing advanced AI features like NLP. Small businesses may find DataRobot or Microsoft Azure more cost-effective for simpler ML needs.
Conclusion
Choosing the right machine learning platform depends on various factors, including project size, budget, and team expertise. For enterprises, Google Cloud AI Platform and IBM Watson Studio provide advanced tools and scalability. For beginners or smaller teams, Microsoft Azure and DataRobot offer accessible interfaces with efficient, automated tools. By weighing each platform’s features, pros, and cons, digital marketing professionals, developers, and content creators can select a platform that aligns with their unique goals and resources.