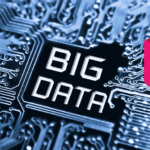
The Role of AI in Big Data
September 14, 2024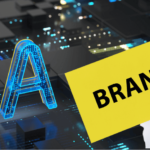
How to Use Artificial Intelligence to Create Stunning Visuals for Your Brand
September 14, 2024In today’s data-driven world, the debate between AI vs Traditional Analytics Which One Is More Efficient continues to captivate professionals. As businesses increasingly rely on insights to drive decision-making, the choice between Artificial Intelligence (AI) and traditional analytics tools has become a crucial consideration. In this article, we’ll explore both approaches, analyze their efficiency, and help you decide which might be the best fit for your business.
Table of Contents
1. What Are Traditional Analytics?
Traditional analytics refers to statistical methods and tools that have been used for decades to analyze historical data. These include techniques like descriptive, diagnostic, and predictive analytics. Traditional analytics rely on human-led processes where analysts define models, extract data, and manually interpret the results.
1.1 Key Methods of Traditional Analytics
- Descriptive Analytics: Summarizes historical data to understand what has happened in the past.
- Diagnostic Analytics: Explores why certain outcomes occurred by analyzing the causes behind data patterns.
- Predictive Analytics: Uses statistical models to predict future outcomes based on past data.
Traditional analytics is often dependent on human input to identify trends, correlations, and patterns. It’s widely used in industries such as finance, retail, and healthcare.
1.2 Pros of Traditional Analytics
- Proven Methods: Traditional analytics tools like Excel, SQL, and SPSS have been trusted for years.
- Easy to Understand: Simpler methods are easier to explain to non-technical stakeholders.
- Low Cost: Requires fewer complex technologies, making it more affordable for small businesses.
1.3 Cons of Traditional Analytics
- Time-Consuming: Analysts need significant time to clean, process, and analyze the data.
- Limited Scalability: Handling massive datasets with traditional tools can be cumbersome.
- Bias Risk: Human-led processes may introduce biases, affecting the accuracy of insights.
2. What is AI-Powered Analytics?
AI-powered analytics refers to using machine learning (ML) and artificial intelligence techniques to automate and enhance the data analysis process. Instead of manually inputting models, AI can identify patterns, make predictions, and recommend actions without human intervention.
2.1 Key Features of AI Analytics
- Automation: AI can automatically cleanse, categorize, and analyze data without manual input.
- Real-time Processing: AI-driven tools can process data in real-time, enabling businesses to react immediately to new information.
- Deep Learning Capabilities: AI’s ability to analyze unstructured data, like images or natural language, allows for broader applications across industries.
2.2 Pros of AI Analytics
- Speed and Efficiency: AI can analyze large datasets in seconds, producing faster insights.
- Accuracy and Precision: AI systems can reduce human error and bias, leading to more reliable outcomes.
- Scalability: AI-driven platforms can handle huge datasets, making them ideal for enterprises dealing with Big Data.
2.3 Cons of AI Analytics
- High Cost: Implementing AI-powered tools can be expensive, requiring significant investment in technology and talent.
- Complexity: AI-driven analytics may be difficult to explain to non-technical teams.
- Data Quality Dependency: AI relies heavily on high-quality data to produce accurate results.
3. Comparing Efficiency: AI vs Traditional Analytics
3.1 Speed and Data Processing
One of the biggest differences between AI and traditional analytics is the speed at which data is processed. Traditional methods often require days or weeks of manual analysis. On the other hand, AI can sift through terabytes of data in a matter of seconds.
- Example: A retail company using traditional analytics may need weeks to analyze consumer data manually. In contrast, an AI-powered system can instantly generate insights about customer preferences, enabling quicker business decisions.
3.2 Scalability
When it comes to scalability, AI-powered analytics far outpaces traditional methods. As businesses accumulate more data, traditional analytics tools struggle to handle the sheer volume. AI, with its ability to process Big Data, provides a scalable solution that grows with the business.
- Statistical Support: According to a study by McKinsey, companies using AI saw a 10-15% increase in productivity due to better scalability.
3.3 Accuracy and Precision
Traditional analytics are prone to human error, whether it’s from incorrect model selection or data misinterpretation. AI systems, in contrast, have the ability to improve their accuracy over time through machine learning models that continuously learn and optimize.
- Real-World Insight: Healthcare companies, for example, benefit from AI in diagnostics. IBM Watson’s AI-driven analytics are known for improving diagnostic accuracy by up to 30%, according to a 2021 study.
3.4 Ease of Implementation
Implementing traditional analytics requires a trained team to handle data cleansing, model building, and interpretation. AI-powered analytics, while more advanced, comes with automated systems that can handle the entire data pipeline. However, the initial setup for AI can be complex, requiring integration with existing infrastructure.
4. Real-World Applications of AI and Traditional Analytics
4.1 Marketing Campaigns
- AI in Action: Marketers use AI-powered tools like HubSpot and Marketo to automate audience targeting, content recommendations, and customer journey mapping. These tools process vast amounts of data to predict customer behavior, optimizing campaigns in real-time.
- Traditional Analytics: Before AI, marketers used descriptive analytics to understand past campaign performance and manually adjusted future strategies.
4.2 E-Commerce
- AI in Action: Amazon’s recommendation engine is powered by AI, providing personalized product suggestions based on user behavior. This has resulted in a significant increase in average order value.
- Traditional Analytics: In the past, e-commerce sites relied on static data such as purchase history to manually recommend products, which lacked personalization.
4.3 Financial Services
- AI in Action: AI-driven fraud detection systems in banks can detect anomalies in transactions and flag suspicious activities instantly, minimizing fraud risks.
- Traditional Analytics: Financial analysts used to manually analyze transaction patterns, which was time-consuming and often missed subtle fraudulent behavior.
5. Choosing the Right Approach: AI or Traditional Analytics?
5.1 Business Size and Resources
Small to medium-sized businesses (SMBs) with limited budgets may opt for traditional analytics, which is easier to implement and doesn’t require significant technological investment. However, enterprises dealing with Big Data and complex processes benefit more from the scalability and speed of AI-powered analytics.
5.2 Industry-Specific Needs
Industries like healthcare, finance, and retail, where real-time data is crucial, find AI-powered analytics indispensable. Meanwhile, industries with simpler data requirements may still find value in traditional methods.
5.3 Cost vs. Benefit
The high cost of implementing AI is a significant barrier for many companies. Traditional analytics remains a cost-effective option for those not ready to invest in AI. However, the long-term gains in efficiency and productivity often outweigh the initial investment in AI.
AI vs Traditional Analytics Which One Is More Efficient, Recap
Feature | AI-Powered Analytics | Traditional Analytics |
---|---|---|
Automation | Fully automated data processing, analysis, and reporting | Manual data cleansing, model building, and interpretation |
Scalability | Handles Big Data effortlessly with real-time processing | Limited to small or medium-sized datasets |
Accuracy | High accuracy through machine learning; improves over time | Prone to human errors and biased model assumptions |
Implementation Cost | High initial investment; cost-effective for large-scale operations | Lower costs, ideal for small businesses |
Ease of Use | Complex setup; requires technical expertise | Relatively simple; accessible to non-technical users |
Best For | Enterprises, industries requiring real-time data analysis | Small businesses, simpler analytical needs |
Frequently Asked Questions (FAQs)
1. What’s the main difference between AI and traditional analytics?
The main difference lies in automation and scalability. AI-powered analytics automates the entire data analysis process and can scale to handle large datasets in real-time, while traditional analytics relies on manual processes and is more limited in scope.
2. Is AI always more accurate than traditional analytics?
Not necessarily. While AI can reduce human error and improve accuracy over time, its effectiveness depends on the quality of the data it’s trained on. Traditional analytics can still be accurate but is more prone to errors from manual processes.
3. Can small businesses benefit from AI-powered analytics?
Yes, but it depends on their needs and resources. Small businesses with complex data and fast-paced environments may benefit from AI’s real-time insights. However, the cost of AI tools can be prohibitive for smaller organizations.
4. How long does it take to implement AI-powered analytics?
The implementation time varies depending on the complexity of the system and the business’s infrastructure. Simple AI tools may take weeks to implement, while more complex systems could take months.
Conclusion
In the debate of AI vs Traditional Analytics: Which Is More Efficient?, both approaches have their merits. AI-powered analytics offers superior speed, scalability, and accuracy, making it the better choice for enterprises dealing with Big Data. Traditional analytics, however, remains a cost-effective and reliable solution for smaller businesses with simpler needs. Ultimately, the right choice depends on your business size, industry, and long-term goals.